How to Solve MATLAB-Based Microgrid System Assignments Using Model Predictive Control
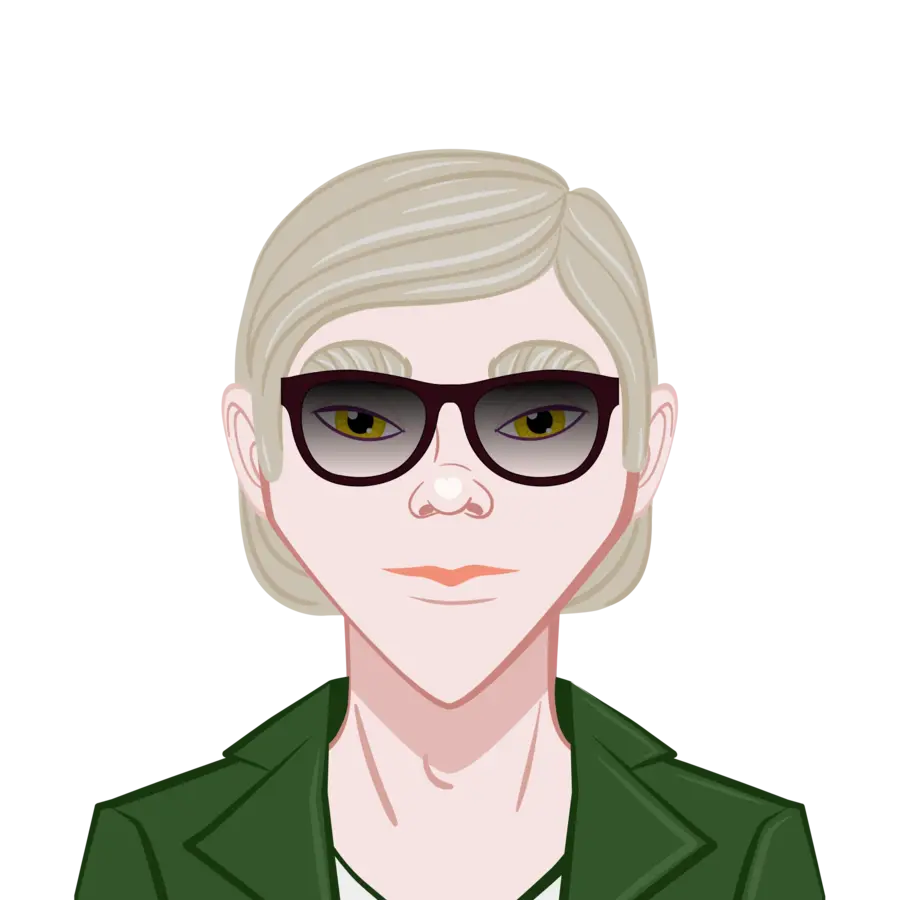
Microgrid systems have become central to modern electrical engineering, particularly in the context of renewable energy integration and efficient power distribution. In assignments involving microgrids, students often encounter complex systems consisting of photovoltaic (PV) panels, energy storage devices such as batteries and supercapacitors, AC generators, and controllers like Model Predictive Control (MPC). These assignments typically require students to use simulation tools like MATLAB/Simulink to model and control various components, ensuring efficient power management and system optimization.
The purpose of this guide is to provide a step-by-step approach for students who are tasked with assignments involving microgrid systems controlled by MPC, as described in the example assignment. This guide will cover how to model and simulate each component of a microgrid system, configure MPC, and interpret simulation results. While the exact details to solve your matlab assignment may differ, the fundamental principles covered in this guide will be applicable to a broad range of similar assignments.
1. Understanding the Microgrid System Components
Before diving into the details of solving the assignment, it’s essential to understand the key components of the microgrid system you are working with. A typical microgrid is an integrated system of various energy sources, storage devices, and loads, all controlled and optimized to work together efficiently. The components you might encounter include:
Photovoltaic (PV) Panels
Photovoltaic panels are renewable energy sources that convert sunlight into electrical power. The output of the PV panel depends on environmental factors such as sunlight intensity and temperature. In your assignment, the PV panel’s output must be modeled accurately to ensure that it integrates correctly with the rest of the system.
When modeling the PV panel, you need to consider the parameters that influence its power generation, including maximum power output, voltage, and the resistance values that govern its performance. These parameters must be carefully adjusted to avoid issues like algebraic loops in the simulation.
Energy Storage Devices: Battery and Supercapacitor
Energy storage devices, including batteries and supercapacitors, play a critical role in balancing the supply and demand within the microgrid. The battery stores energy when there is surplus power and provides energy to the system when demand exceeds the available generation. The supercapacitor, on the other hand, provides quick bursts of power during peak demand.
The state of charge (SOC) of both the battery and supercapacitor must be tracked over time, as this determines their available energy. In the case of the battery, the charging and discharging cycles are governed by the system's requirements, while the supercapacitor typically responds to high-power demands quickly.
Synchronous Machine (Generator)
The synchronous machine in the microgrid acts as an AC generator. It is typically controlled to match the load requirements of the system. The synchronous machine should be modeled to ensure that its output voltage and frequency are aligned with the system’s AC grid.
Model Predictive Control (MPC)
Model Predictive Control (MPC) is a type of control strategy that uses a model of the system to predict future states and optimize control actions. The MPC operates by solving an optimization problem at each time step, adjusting the control inputs to minimize a predefined cost function while ensuring that the system’s constraints are respected.
In microgrid systems, the MPC typically manages the power output of various components, such as the load, synchronous machine, and supercapacitor, to ensure that the energy supply meets the demand efficiently. The MPC uses internal models of the energy storage devices (battery and supercapacitor) to predict their future states, making real-time adjustments based on these predictions.
2. Modeling the System Components in MATLAB
Once you understand the system components, the next step is to model them in MATLAB/Simulink. The complexity of the system requires an accurate representation of each component, including the PV panel, battery, supercapacitor, synchronous machine, and MPC. Below are the key steps involved in modeling these components.
Modeling the Photovoltaic (PV) Panel
The PV panel’s power output is primarily determined by the available solar radiation and the efficiency of the panel. To model the PV panel in MATLAB, you need to consider both the electrical characteristics of the panel and the impact of varying environmental conditions.
- Defining the Power Output: The power output of the PV panel can be calculated using a mathematical model that depends on the irradiance (sunlight intensity) and temperature. The PV panel’s output is typically expressed as a function of these variables, with parameters such as the maximum power (Pmax) and voltage (V) used to define the panel’s behavior.
- Adjusting the Resistance: The resistance in the circuit connected to the PV panel must be set carefully to avoid algebraic loop errors. In some assignments, like the one described, the resistance may be optimized to ensure that the PV panel’s output is maximized under different irradiation levels.
Tip: Ensure that you select the correct resistance range to avoid issues with the simulation. Adjusting resistance impacts the voltage and current characteristics of the panel, which in turn affects the system’s power output.
Modeling the Battery and Supercapacitor
The battery and supercapacitor are typically modeled using state-space representations, where the state variables represent their SOC. The SOC of the battery and supercapacitor evolves over time based on charging and discharging rates.
- State-Space Representation: A state-space model can represent the dynamics of the battery and supercapacitor. The equations governing their SOC are typically first-order differential equations that describe how the battery and supercapacitor charge and discharge based on the input power.
- Controller for Supercapacitor: While the MPC handles the overall system optimization, the supercapacitor might be controlled by a PI (Proportional-Integral) controller. The reference value for the PI controller is provided by the MPC, ensuring that the supercapacitor discharges during peak demand and charges when there is excess power.
Modeling the Synchronous Machine
The synchronous machine is modeled to simulate its operation as an AC generator. This component is controlled by the MPC to match the system’s requirements. The machine’s behavior is typically modeled using electrical circuit equations, with control inputs adjusting the machine’s output power.
Configuring the Model Predictive Controller (MPC)
The MPC uses a model of the battery and supercapacitor (state-space model) to predict the system's future states and optimize control inputs. The key steps involved in configuring the MPC include:
- Plant Model: The plant model of the MPC represents the system dynamics, including the battery and supercapacitor. The MPC uses this model to predict the future states of the system and calculate the necessary control actions.
- Optimization Problem: At each time step, the MPC solves an optimization problem, where the control inputs (e.g., load power, supercapacitor power, synchronous machine power) are adjusted to minimize a cost function. The cost function could represent energy efficiency, stability, or other performance metrics.
- Internal Plant Model and Parameters: The internal plant model of the MPC includes matrices that represent the state-space model of the system. In your assignment, you need to determine the best values for parameters like K1 and K2, which affect the dynamics of the plant model.
Tip: Use MATLAB’s optimization toolbox to find the best values for the MPC parameters. You may need to adjust the signs of certain terms in the matrices (e.g., B, D, Dd) to achieve the best performance.
3. Simulating the System and Analyzing the Results
Once you have modeled the system components and configured the MPC, the next step is to simulate the system and analyze the results. Here are the key steps involved in simulation:
Running the Simulation
Run the simulation to observe the behavior of the system over time. You should focus on the following key aspects:
- State of Charge (SOC) of Battery and Supercapacitor: The SOC of the battery and supercapacitor will change over time as the system charges and discharges these storage devices. Track how the SOC evolves in response to load changes, PV output variations, and MPC control actions.
- Manipulated Variables: The MPC adjusts several control variables, such as load power, supercapacitor power, and synchronous machine power. Plot these manipulated variables to see how the MPC adjusts the system’s inputs to optimize performance.
- Cost Function: The MPC aims to minimize the cost function, which typically represents system efficiency or stability. After running the simulation, examine how the cost function evolves. In some cases, removing the PV parameter from the simulation should result in a higher cost, indicating that the PV panel is essential for maintaining an efficient system.
Visualizing the Results
Use MATLAB’s plotting functions to visualize the results of the simulation. Common plots to generate include:
- SOC Plots: Plot the SOC of the battery and supercapacitor over time. This will help you understand when the supercapacitor is being used (e.g., during peak load periods).
- Manipulated Variable Plots: Plot the manipulated variables (e.g., Pload, Psc, Psm) to visualize how the MPC adjusts them in real-time.
- Cost Function Plot: Plot the cost function to see how it is minimized over time. This will help you assess the performance of the MPC.
Interpreting the Results
Analyze the plots to assess the system’s performance. Pay attention to the following:
- Supercapacitor Usage: Identify the times when the supercapacitor is used most frequently. This typically occurs during high peak load periods when quick bursts of power are needed.
- MPC Effectiveness: Evaluate how well the MPC is able to maintain system stability and minimize the cost function. If the cost function is significantly higher without the PV panel’s input, it indicates the importance of renewable energy in the microgrid.
4. Troubleshooting Common Issues
When working with complex systems like this, you may encounter several common issues:
- Algebraic Loops: Algebraic loops occur when variables are dependent on each other in a way that causes circular dependencies. Ensure that you set up the resistance parameters correctly and avoid feedback loops that cannot be solved.
- Optimization Convergence: The MPC uses optimization algorithms to determine the best control actions. Sometimes, the optimization problem may fail to converge. Try adjusting the initial conditions, tuning the MPC parameters, or increasing the solver's tolerance to improve convergence.
- Inconsistent System Behavior: If the system behaves unexpectedly, check your models for errors in parameter values or control algorithms. Use MATLAB’s debugging tools to identify and fix issues in the simulation.
Conclusion
Successfully solving a microgrid system assignment using Model Predictive Control (MPC) in MATLAB requires a deep understanding of both the system components and the control strategy. Throughout this guide, we have explored the key elements of a microgrid, including photovoltaic (PV) panels, energy storage devices like batteries and supercapacitors, and synchronous machines. We have also detailed the process of modeling these components in MATLAB/Simulink, configuring the MPC to optimize system performance, and analyzing the simulation results.