How to Solve MATLAB Assignments on Model Predictive Control (MPC)
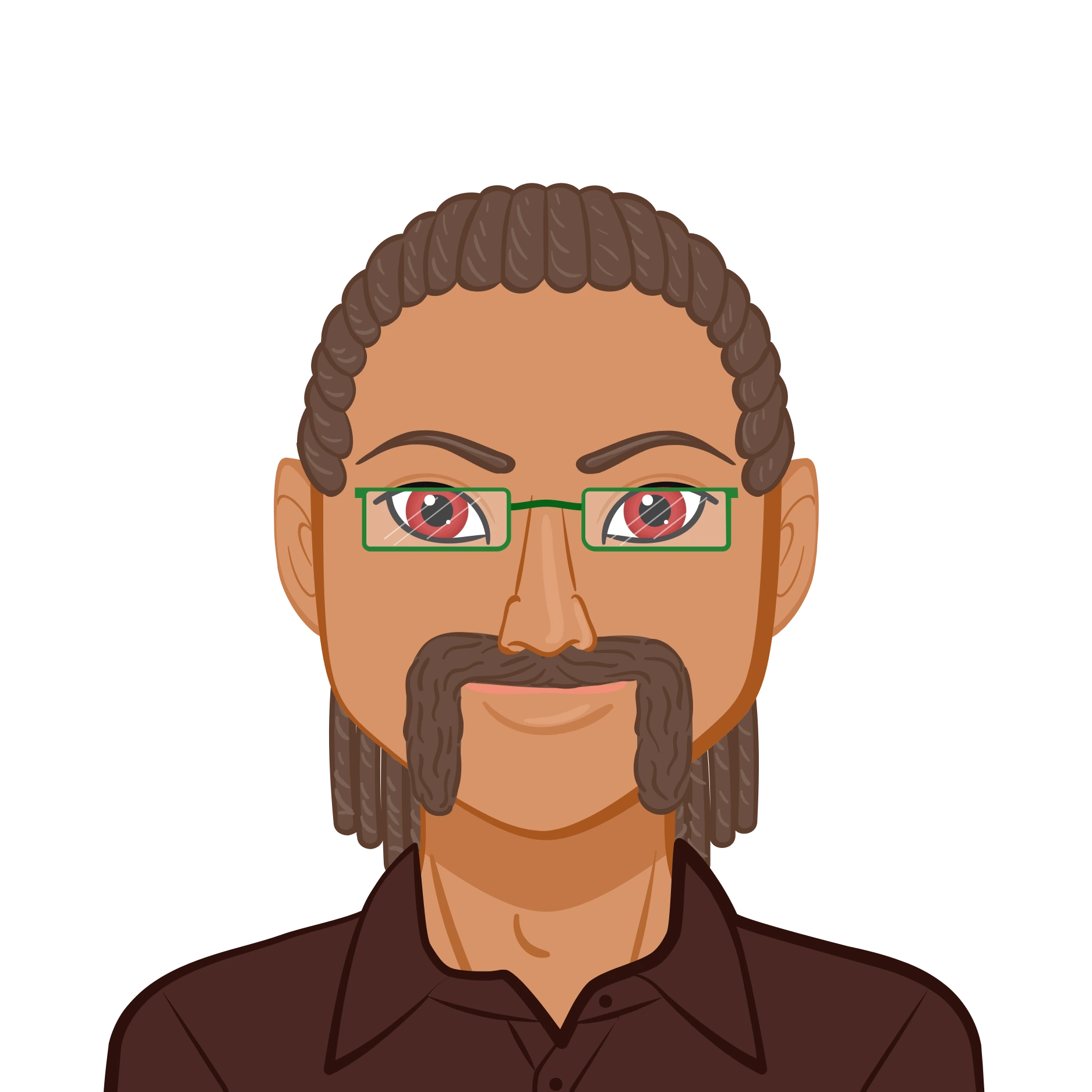
Model Predictive Control (MPC) is a powerful control strategy widely applied in industries such as automotive, aerospace, and process control. It determines optimal control actions by solving a constrained finite-horizon optimization problem at each time step. Understanding its formulation, implementation, and analysis is essential for students working on MPC assignments. MATLAB is one of the most effective tools for designing and simulating MPC controllers due to its built-in optimization functions and toolboxes like Model Predictive Control Toolbox.
When implementing MPC in MATLAB, students need to define system dynamics using state-space models, formulate an appropriate cost function, and apply constraints to ensure feasibility. MATLAB’s fmincon function can be used to solve constrained optimization problems iteratively, adjusting control inputs dynamically. The software also allows visualization of system response through plots, helping in performance evaluation. For example, in a robotic path-tracking problem, MPC can optimize wheel velocities while avoiding obstacles. Similarly, in a temperature control system, MATLAB can model heat transfer dynamics and compute optimal heating power to maintain the desired temperature. By leveraging MATLAB’s simulation capabilities, students can analyze MPC behavior under different conditions, improving their understanding of constraint handling and stability in real-world applications. This guide provides a structured approach how to solve your Matlab assignment on Model Predictive Control (MPC) effectively.
Understanding the Assignment Requirements
Before starting an MPC assignment, carefully review the guidelines and assessment criteria. These typically require demonstrating knowledge of advanced control techniques, translating performance specifications into functional requirements, designing and implementing an MPC controller, and evaluating its performance. A structured approach ensures efficiency and accuracy in solving the problem.
A strong foundation in control theory is crucial. Techniques such as Linear Quadratic (LQ) optimal control, Proportional-Integral-Derivative (PID) controllers, and state-space modeling play a key role in formulating and implementing MPC. Understanding system dynamics, constraints, and defining an appropriate cost function are essential for achieving optimal control performance.
MATLAB is a powerful tool for implementing MPC controllers. It provides built-in functions, such as the mpc toolbox, which simplifies the design process. However, if toolbox restrictions apply, coding the optimization problem manually using functions like fmincon or quadratic programming solvers is necessary. MATLAB’s simulation capabilities help in validating the controller by testing it under different conditions.
To ensure success, structuring the problem correctly, tuning parameters effectively, and debugging systematically are vital. Running multiple simulations and analyzing controller behavior using MATLAB plots enhances understanding. By following a methodical approach, students can develop robust MPC controllers and gain practical insights into real-world applications.
Formulating the MPC Optimization Problem
One of the first steps is to construct the constrained finite-horizon optimal control problem. This involves defining state-space models, specifying constraints, and selecting a prediction horizon. The cost function must balance tracking performance with control effort, ensuring the controller is both effective and efficient. Choosing the right weight values for the cost function significantly impacts the system's behavior.
Example: Temperature Control System
Consider an industrial temperature control system where the goal is to maintain the temperature at 100°C while minimizing energy consumption. The state-space model includes temperature as the state variable and heater power as the control input. Constraints ensure that the heater operates within safe limits. The cost function penalizes large temperature deviations and excessive energy usage, striking a balance between precision and efficiency.
Tuning the MPC controller requires careful parameter selection, including prediction and control horizons. Effective constraint handling is essential, ensuring that inputs and states remain within the desired range. MATLAB can be used to solve the optimization problem, but if the use of toolboxes is restricted, coding the solution from scratch is necessary.
MATLAB Code for System Definition:
A = 1; % System dynamics matrix
B = 0.1; % Input matrix
C = 1; % Output matrix
D = 0; % Direct transmission matrix
x0 = 20; % Initial temperature
Ts = 1; % Sampling time
sys = ss(A, B, C, D, Ts);
Implementing the MPC Controller in MATLAB
Once the problem is defined, the next step is writing MATLAB scripts to implement the controller. This involves solving the constrained optimization problem iteratively at each time step. Structuring the code efficiently using functions and loops can help in organizing the implementation. Running simulations under different initial conditions and disturbance scenarios tests the robustness of the controller.
Example: Robot Path Tracking
Imagine a mobile robot navigating a predefined path while avoiding obstacles. The MPC controller determines the optimal wheel velocities to follow the path smoothly while maintaining stability. Constraints prevent excessive acceleration that could cause slipping. The MATLAB implementation involves defining the robot’s kinematic model, specifying state and input constraints, and simulating performance under various conditions.
Generating labeled plots for state trajectories, control inputs, and cost function values is crucial for performance evaluation. Analyzing these results helps identify stability, feasibility, and offset-free tracking performance, providing valuable insights into controller behavior.
MATLAB Code for MPC Implementation:
mpcHorizon = 10; % Prediction horizon
Q = 10; % State weight
R = 1; % Control weight
% Define constraints
umin = -1; umax = 1; % Control limits
xmin = -5; xmax = 5; % State limits
% Solve optimization iteratively
for k = 1:100
% Define cost function and constraints
J = x(k)^2 * Q + u(k)^2 * R;
constraints = [umin <= u(k) <= umax, xmin <= x(k) <= xmax];
u(k) = fmincon(@(u) J, 0, [], [], [], [], umin, umax);
x(k+1) = A * x(k) + B * u(k);
end
Common Challenges in MPC Assignments
Students often encounter difficulties in selecting an appropriate horizon length. A short horizon may result in aggressive control actions, while a long horizon can increase computational complexity. Striking the right balance ensures optimal performance. Another common issue is tuning weight matrices. Improper selection can lead to excessive control effort or poor tracking, necessitating a structured approach to weight tuning.
Handling constraints effectively is another critical aspect. The optimization algorithm must enforce constraints while maintaining stability. MATLAB implementation errors, such as incorrect indexing or optimization function misuse, can be avoided by systematically debugging the code. Testing individual components separately before integration is a good practice to follow.
Example: Autonomous Vehicle Speed Control
Consider an autonomous car that adjusts its speed based on traffic conditions. The MPC controller ensures smooth acceleration and braking while respecting speed limits and maintaining a safe following distance. Implementing this in MATLAB involves setting up a cost function that minimizes speed deviations while penalizing sudden changes in acceleration.
MATLAB Code for Speed Control:
N = 20; % Prediction horizon
v_ref = 30; % Desired speed (m/s)
Q = 100; % Speed tracking weight
R = 1; % Control effort weight
% Initialize variables
v = zeros(1, N);
u = zeros(1, N);
for k = 1:N
J = (v(k) - v_ref)^2 * Q + u(k)^2 * R;
u(k) = fmincon(@(u) J, 0, [], [], [], [], -5, 5);
v(k+1) = v(k) + u(k) * 0.1; % Vehicle dynamics
end
plot(1:N, v, '-b'); xlabel('Time Step'); ylabel('Speed (m/s)');
title('MPC Speed Control');
grid on;
Best Practices for Solving MPC Assignments
Solving MPC assignments effectively requires a structured approach. Breaking the problem into smaller tasks, validating results through multiple test cases, and analyzing controller behavior under different conditions are key practices. A strong theoretical foundation in MPC principles, including constraint handling and stability considerations, is essential. Debugging MATLAB implementations systematically and testing individual components before integration help ensure accuracy. Simulating real-world scenarios and interpreting performance metrics enhance understanding and improve problem-solving skills.
Conclusion
Mastering MPC assignments requires a strong conceptual foundation, structured problem-solving techniques, and proficiency in MATLAB implementation. By understanding system dynamics, formulating a well-structured optimization problem, and effectively tuning controller parameters, students can develop robust MPC controllers. Simulating various scenarios and analyzing performance comprehensively strengthens the ability to design and evaluate advanced control systems. With consistent practice and a methodical approach, tackling MPC assignments becomes a more manageable and rewarding experience.