How to Address MATLAB Assignment on Computer Vision and Robotic Arm Integration
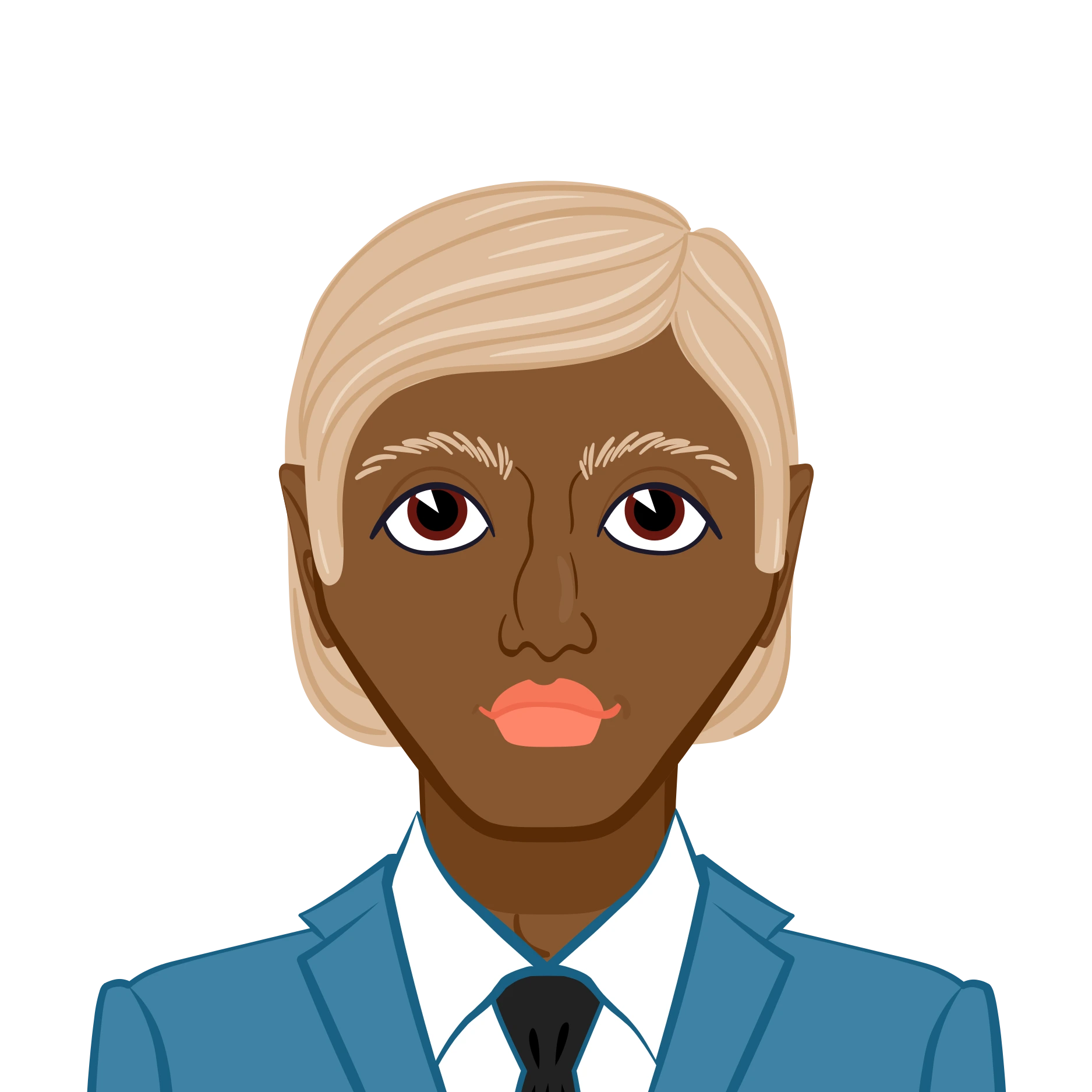
MATLAB is a powerful tool for solving complex engineering problems, especially in fields like computer vision and robotics. One of the most fascinating applications of MATLAB is integrating a robotic arm with computer vision to perform automated tasks such as pick-and-place operations. This blog will guide students on how to approach similar MATLAB assignments by breaking down the essential steps and providing insights into problem-solving techniques.
Understanding how to use image processing techniques, homography transformation, and motion planning algorithms like Bug2 can help students to complete their Matlab assignment related to robotic and computer vision systems. This guide will help students tackle MATLAB assignments involving object identification, pose estimation, and path planning using real-world examples.
Understanding the Problem Statement
Before jumping into coding, it is crucial to break down the problem statement into clear objectives. Many MATLAB assignments in robotics and computer vision involve:
- Identifying the game board and its reference position to the robotic arm.
- Detecting and classifying objects based on color.
- Calculating real-world coordinates using image transformation techniques.
- Implementing path planning algorithms like Bug2 to navigate a game board.
- Handling dynamic changes and making real-time adjustments.
A clear understanding of these concepts will make solving the assignment more structured and manageable.
Setting Up MATLAB for Computer Vision Tasks
To successfully solve a MATLAB assignment involving robotic vision, students must first configure their MATLAB environment with the necessary toolboxes. The following steps will help set up MATLAB for computer vision and robotic arm programming:
- Install Required Toolboxes – MATLAB’s Computer Vision Toolbox and Robotics Toolbox are essential for image processing and robotic arm control.
- Connect the Webcam – Use MATLAB’s built-in webcam function to capture live images.
- Calibrate the Camera – Use the estimateCameraParameters function to correct distortions and obtain accurate measurements.
Once these steps are completed, students can begin processing images and detecting objects on the game board.
Identifying the Game Board and Objects
Identifying the game board and objects is the first crucial step in solving a MATLAB assignment involving computer vision and robotics. Using a top-down view from a webcam, students must detect the four corners of the game board to establish a reference frame. This requires applying image processing techniques such as edge detection and contour finding. Additionally, object identification is performed using color segmentation, where different colors represent obstacles and the player piece. Once identified, the positions of these objects must be mapped relative to the robot’s base joint using homography transformation, ensuring accurate movement and interaction with the environment.
Capturing and Processing the Image
The first step in object identification is capturing an image from a webcam and processing it. MATLAB provides various functions to read and manipulate images:
cam = webcam;
img = snapshot(cam);
imshow(img);
After capturing the image, the next step is converting it to grayscale and applying thresholding techniques to identify objects.
grayImg = rgb2gray(img);
binaryImg = imbinarize(grayImg);
imshow(binaryImg);
Detecting Game Board Corners
A crucial step in computer vision assignments is detecting the four corners of the game board. This helps in mapping the board’s coordinates to the real-world environment.
Using edge detection and corner detection techniques in MATLAB, students can extract the key features:
edges = edge(grayImg, 'Canny');
corners = detectHarrisFeatures(edges);
imshow(img); hold on;
plot(corners.Location(:,1), corners.Location(:,2), 'r*');
This method provides the real-world coordinates of the board, which are essential for robotic movements.
Homography Matrix and Perspective Transformation
A homography matrix is essential for aligning an image’s perspective with the real-world coordinate system of a game board. It is a 3×3 transformation matrix that maps points from one plane to another, correcting distortions caused by perspective differences. In MATLAB, homography is computed using corresponding points between the image and real-world board. This transformation ensures accurate localization of objects, enabling precise robotic movements. By applying the homography matrix, students can convert the top-down webcam view into a geometrically accurate representation, allowing the robotic arm to navigate and interact with objects effectively within the game board’s coordinate system. This transformation ensures that object positions are accurately mapped.
Calculating the Homography Matrix
To perform a perspective transformation, students must define corresponding points between the image and real-world coordinates. MATLAB’s fitgeotrans function helps in computing the transformation matrix:
imagePoints = [x1, y1; x2, y2; x3, y3; x4, y4];
worldPoints = [X1, Y1; X2, Y2; X3, Y3; X4, Y4];
tform = fitgeotrans(imagePoints, worldPoints, 'projective');
Applying this transformation will map all detected objects to real-world coordinates.
Object Detection Using Color Segmentation
Color segmentation is a widely used technique in computer vision to identify and differentiate objects based on their colors. In the game board scenario, each piece is color-coded, making it easier to segment them using MATLAB. By converting the image into different color spaces like HSV or LAB, color thresholds can be applied to isolate specific objects. Functions like imbinarize() and regionprops() help in detecting object locations. Morphological operations such as dilation and erosion refine the segmentation process, reducing noise. This approach ensures accurate identification of the player piece, obstacles, and board corners, enabling precise robotic movements. MATLAB’s HSV color space provides an effective way to differentiate objects based on color.
hsvImg = rgb2hsv(img);
blueMask = (hsvImg(:,:,1) > 0.5) & (hsvImg(:,:,1) < 0.7);
imshow(blueMask);
This approach allows students to detect obstacles and the player piece efficiently.
Implementing the Bug2 Algorithm for Path Planning
Once the board setup and object detection are complete, the next step is moving the robotic arm using the Bug2 Algorithm. Bug2 is a path-planning algorithm that helps navigate obstacles while reaching a destination.
Understanding the Bug2 Algorithm
The Bug2 algorithm follows these steps:
- Move in a straight line towards the goal.
- If an obstacle is encountered, follow its boundary until a clear path is found.
- Resume the direct path towards the goal.
Implementing Bug2 in MATLAB
To implement Bug2, students must define a grid representation of the board and write a function to handle movement logic:
grid = [1 1 1 1 1;
1 0 0 0 1;
1 0 1 0 1;
1 0 3 0 1;
1 1 1 1 1];
[playerRow, playerCol] = find(grid == 3);
goalRow = 5;
goalCol = 3;
while playerRow ~= goalRow || playerCol ~= goalCol
if grid(playerRow+1, playerCol) == 0
playerRow = playerRow + 1;
elseif grid(playerRow, playerCol+1) == 0
playerCol = playerCol + 1;
end
grid(playerRow, playerCol) = 3;
end
This algorithm helps the robotic arm find a clear path and reach its destination.
Handling Dynamic Changes and Obstacle Adjustments
In real-world scenarios, obstacles may shift unexpectedly, requiring real-time adjustments to ensure smooth robotic operations. A robust system must continuously monitor changes using computer vision and update the robot’s path accordingly. By implementing dynamic obstacle detection and adaptive path planning, such as the Bug2 algorithm, the robot can navigate efficiently while avoiding collisions. MATLAB’s image processing and path-planning capabilities enable real-time responses, ensuring the robot adapts to environmental changes. Handling such dynamic conditions enhances the system’s reliability, making it more suitable for real-world applications like industrial automation, warehouse robotics, and autonomous navigation in unpredictable environments. MATLAB’s object tracking functions, such as vision.PointTracker, help monitor changes dynamically.
tracker = vision.PointTracker('MaxBidirectionalError', 2);
initialize(tracker, corners.Location, img);
[newPoints, validity] = tracker(img);
By integrating tracking with movement logic, students can ensure their robotic system adapts to changes effectively.
Advanced Optimization with Inverse Kinematics
To enhance motion control in robotic systems, students can apply inverse kinematics for smoother and more precise trajectories. Instead of relying on predefined joint movements, inverse kinematics calculates the optimal joint angles needed to reach a specific end position. This approach minimizes abrupt changes in motion, ensuring continuous and fluid transitions between waypoints. By integrating inverse kinematics in MATLAB, students can improve the efficiency of robotic arm movements, reduce unnecessary joint stress, and achieve better accuracy in pick-and-place operations. Mastering this technique is essential for applications in automation, robotics research, and AI-driven vision systems. MATLAB’s inverseKinematics function computes the best joint angles for desired positions.
ik = inverseKinematics('RigidBodyTree', robot);
jointAngles = ik('end_effector', trvec2tform([x, y, z]), [1 1 1 1 1 1]);
This method ensures precise and efficient movement of the robotic arm.
Conclusion
Solving MATLAB assignments involving robotic arms and computer vision requires a structured approach and a solid understanding of key concepts. To effectively solve their computer vision systems assignment, students should break down the problem into manageable tasks, ensuring a clear workflow. Setting up MATLAB with the necessary toolboxes, such as the Computer Vision Toolbox and Robotics System Toolbox, is crucial for processing images and controlling robotic movements. Object detection using color segmentation and applying homography transformations for accurate positioning are fundamental steps.
Implementing path planning algorithms like Bug2 and adapting to dynamic changes help the robotic system navigate efficiently in real-world environments. Additionally, integrating inverse kinematics for smoother motion control enhances precision in robotic arm operations, making movements more efficient and reducing abrupt transitions. These techniques not only improve assignment performance but also provide valuable hands-on experience in automation, AI-driven vision systems, and robotics. By mastering these skills, students can gain knowledge applicable to industrial applications and research in autonomous robotics.